Volume 4 - Year 2023 - Pages 01-05
DOI: 10.11159/jmids.2023.001
Practical Representations of Copula and Joint Density Estimates
Yishan Zang1, Serge Provost1
1The University of Western Ontario, Department of Statistical and Actuarial Sciences
1151 Richmond Street, London, Ontario, Canada N6A 3K7
yzang8@uwo.ca; provost@uwo.ca
Abstract - A moment-based approximation methodology for estimating a copula density from bivariate observations is introduced. The resulting simple representation of the copula density is suitable for reporting purpose or carrying out further algebraic manipulation. Empirical copula density functions will also be determined from kernel density estimates. A technique for obtaining a joint density from marginal density estimates and a copula density is proposed as well. The Bernstein copula density approximants will be utilized for comparison purposes. The results are applied to two stocks’ closing prices and a stock’s price and its running maximum. In the latter case, the model is related to a Brownian motion process.
Keywords: Empirical copulas, Bivariate density estimation, Data modelling, Brownian motion process, Financial application.
© Copyright 2023 Authors - This is an Open Access article published under the Creative Commons Attribution License terms. Unrestricted use, distribution, and reproduction in any medium are permitted, provided the original work is properly cited.
Date Received:2022-06-29
Date Revised: 2023-05-18
Date Accepted: 2023-06-24
Date Published: 2023-07-05
1. Introduction
Copulas are principally utilized for modelling dependencies in multivariate distributions. Their properties have been increasingly exploited in numerous types of scientific investigations; for instance, the reader may refer to Chao et al. [1], Carrera et al. [2] and Chen et al. [3] for recent advances in the area of artificial intelligence. The key idea behind copulas is that the joint distribution of two or more variables can be represented in terms of their marginal distributions and a specific correlation structure. As a measure of dependence, they have for instance found applications in reliability theory, signal processing, geodesy, hydrology and medicine. Results involving empirical bivariate copula densities are discussed in this paper.
Let F(x1,x2 ) be the joint cumulative distribution function of random variables X1 and X2 having continuous marginal distribution functions F1 (x1) and F2 (x2 ). According to Sklar [4], there exists a unique bivariate copula C:I2↦I (the unit interval) such that

where C(⋅,⋅) is a joint cumulative distribution function having uniform marginals. Conversely, for any continuous cumulative distribution function F1 (x1 ) and F2 (x2 ) and any copula C, the function F defined in Equation (1) is a joint distribution function with marginal distributions F1 and F2.
Sklar's theorem provides a technique for constructing copulas. Indeed, the function

is a bivariate copula, where the quasi-inverse Fi(-1) for i=1,2 is defined by

We shall denote the probability density function corresponding to the copula C(u1,u2 ) by

The following relationship between the joint density function f(⋅,⋅) and the copula density function c(⋅,⋅) can readily be obtained from Equation (1):

where f1 (x1 ) and f2 (x2 ) respectively denote the marginal density functions of X1 and X2. Accordingly, the copula density function can be expressed as follows:

The proposed approaches to estimating copula density functions are summarized in Sections 2 and 3. The estimation of a joint density function in terms of marginal distributions and a copula density estimate is discussed in Section 4. Three illustrative examples are provided in the last section.
2. Copula Density Based on Kernel Density Estimation
On applying the kernel density estimation (kde) method to a given bivariate dataset, one can obtain an estimate of the bivariate probability density of X. In light of Equation (6), the copula density can be represented as follows:

where fX can be estimated by a bivariate kde and QXi (⋅) denotes the quantile function. The marginal density function of each variable can be obtained by determining their respective kernel density estimates. The inverse cdfs QX1and QX2 can be determined in polynomial form by making use of a moment-based method or the method of least squares.
3. A Moment-based Bivariate Polynomial Approximation of the Copula Density
Once a copula density is determined from Equation (7), it can be approximated by the product of a base density and a bivariate polynomial whose coefficients are obtained from the joint moments associated with the copula density.
The proposed procedure for achieving this is described in the next result.
Result 1 Let fX (x1,x2 ) be the density function of a bivariate continuous random vector X=(X1,X2 ) defined in the rectangle (l1,u1 )×(l2,u2 ), whose joint moments are

Let ψ(x1,x2) be an initial joint density estimate, whose joint moments are

Assuming that the sequence μX (i,j), i=0,1,2,…, j=0,1,2, uniquely defines the distribution of X, the density function of X can be approximated by

where ξi,j can be determined by letting

h=0,1,…,n;g=0,1,…,n, or equivalently,

h=0,1,…,n; g=0,1,…,n. Thus, we can obtain the polynomial coefficients ξi,jand fn (x1,x2 ) from the moments of fX (⋅) and ψ(⋅) by solving the linear system specified by Equation (12).
The degree n used in the polynomial adjustment should be selected so that fn provides an accurate approximation to the estimate of the copula density, which can be determined for instance by evaluating their integrated squared differences.
4. Estimating a Joint Density from the Marginal Density Estimates and the Copula Density
On applying Equation (5), that is,

one can determine the joint density where for instance, c(⋅,⋅) could be taken as a Bernstein copula density which is described in Sancetta and Satchell [5].
Suppose that observations are available on the random variables X1 and X2. The marginal densities f1 (x1 ) and f2 (x2) are estimated and a Bernstein's copula density of high order is determined. A joint density estimate of (X1,X2) can then be obtained via Equation (5).
5. Applications
The results are applied to two stocks’ closing prices as well as a stock’s price and its running maximum.
5.1. Copula Density Estimation Methodologies Applied to Two Stocks
The two stocks selected are GOOG (Alphabet Inc.) and AAPL (Apple Inc.). The bivariate data are the daily closing prices of (GOOG, AAPL) in 2019. Each component of the data has been standardized. The joint KDE of X, the marginal densities of X1 and X2 and the corresponding cdf inverses are displayed in Figures 1-5.
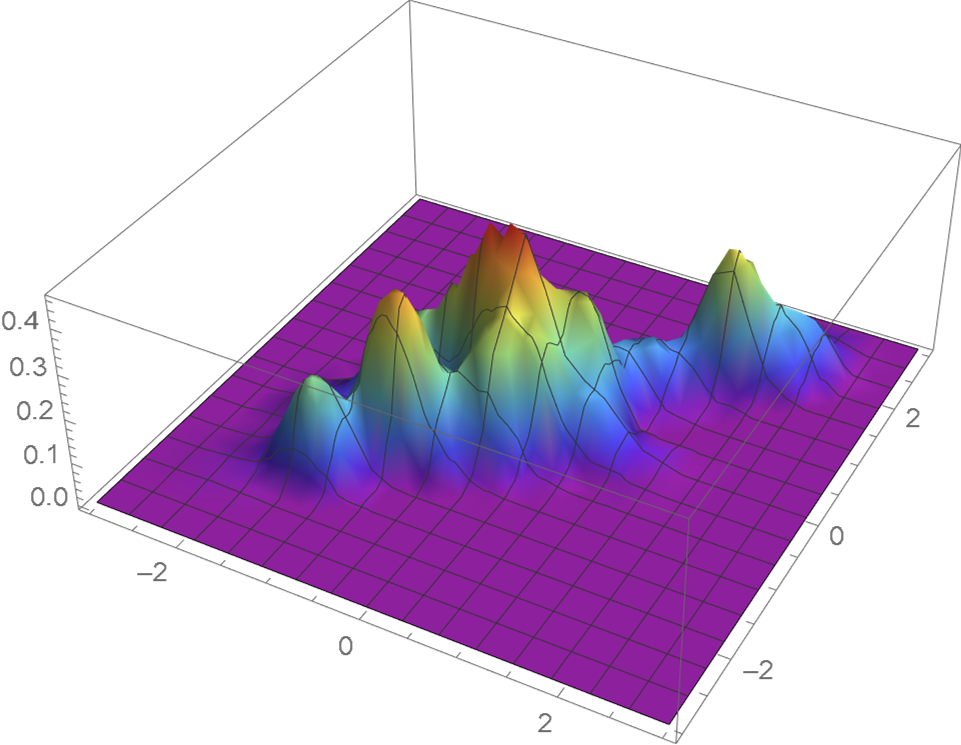
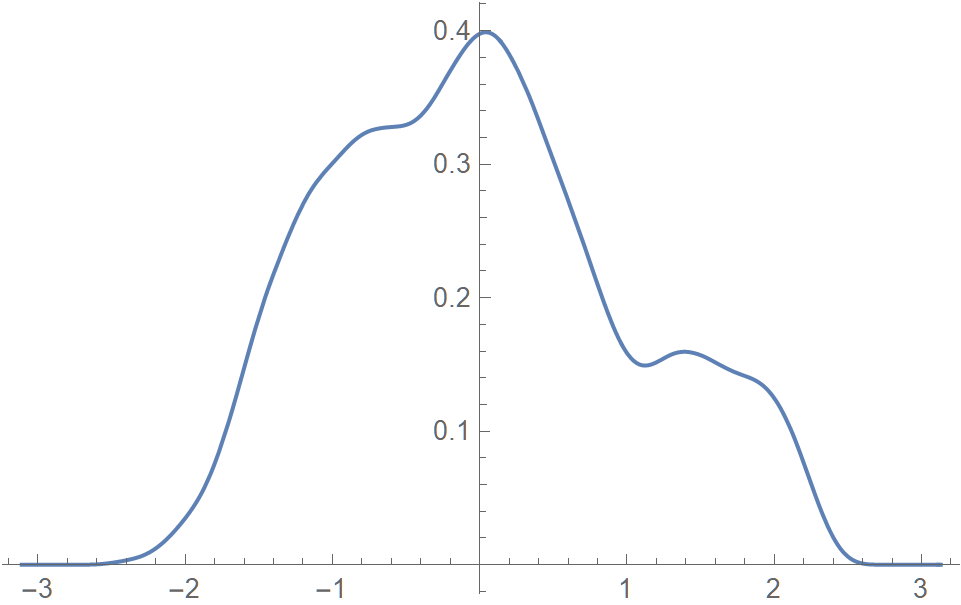
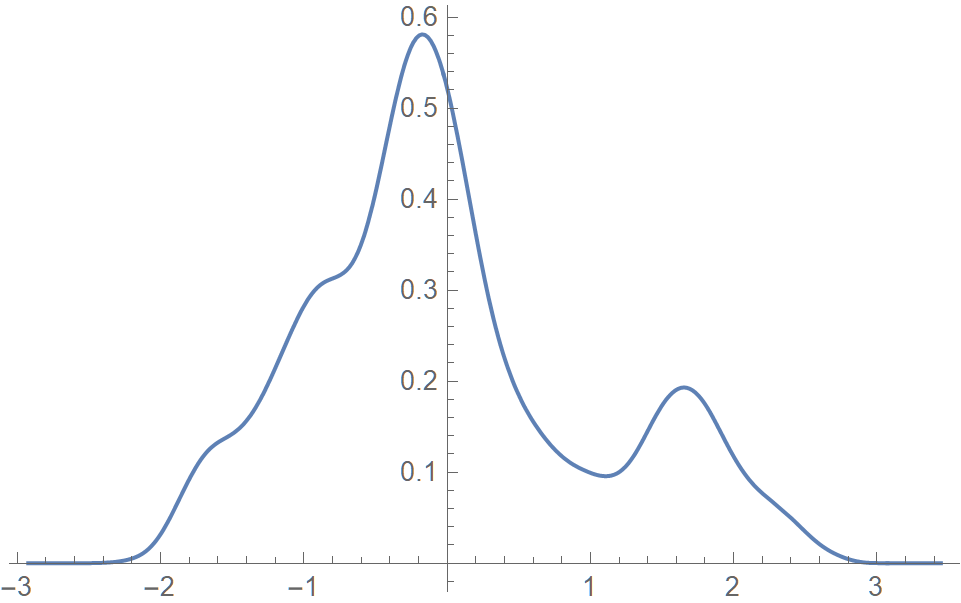
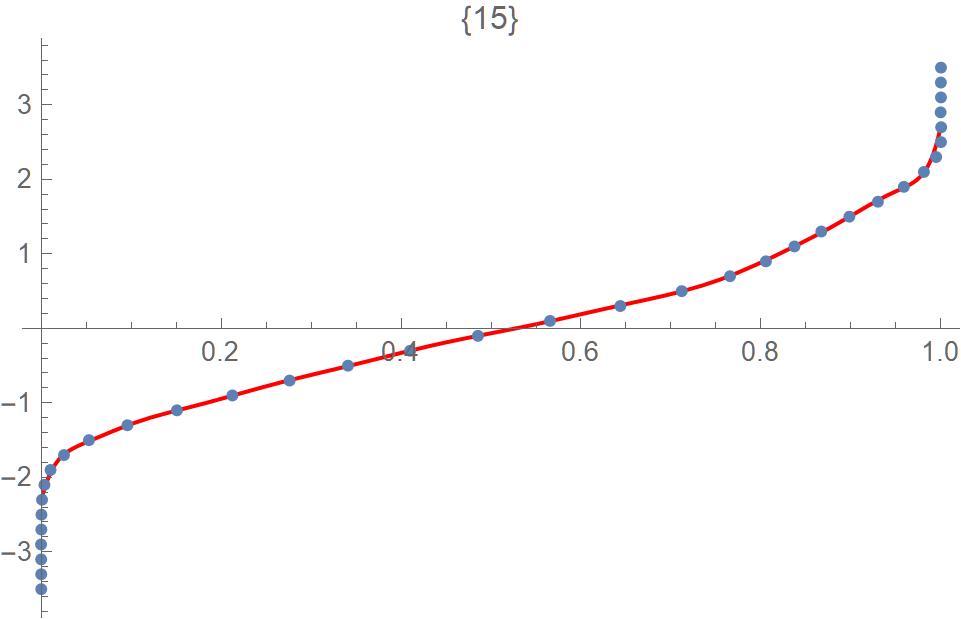

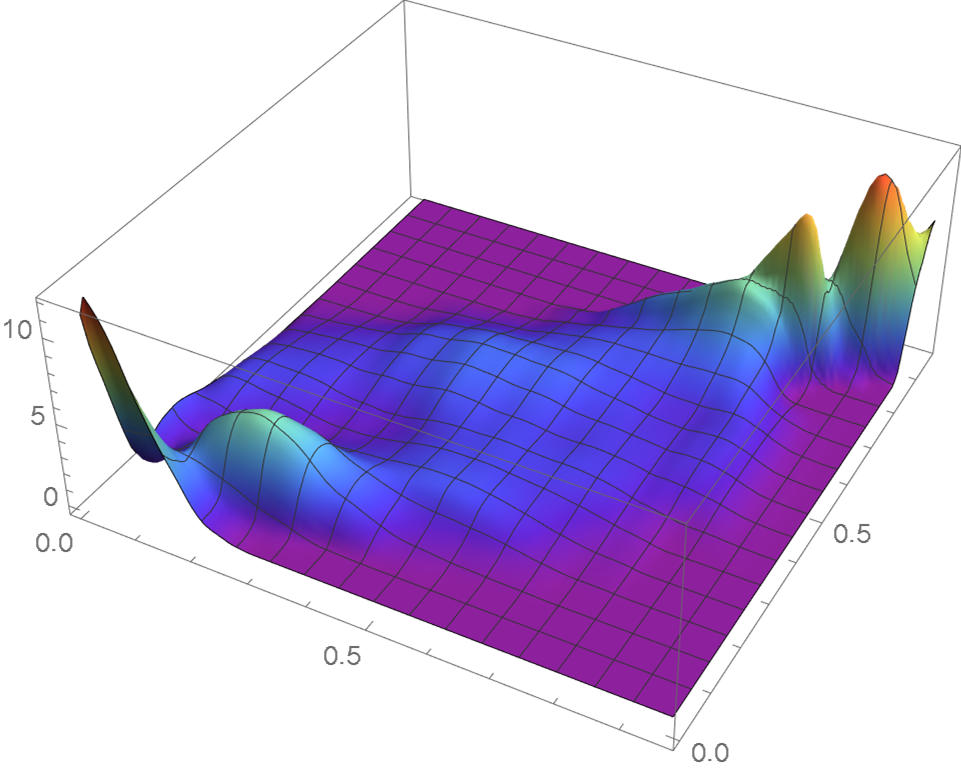
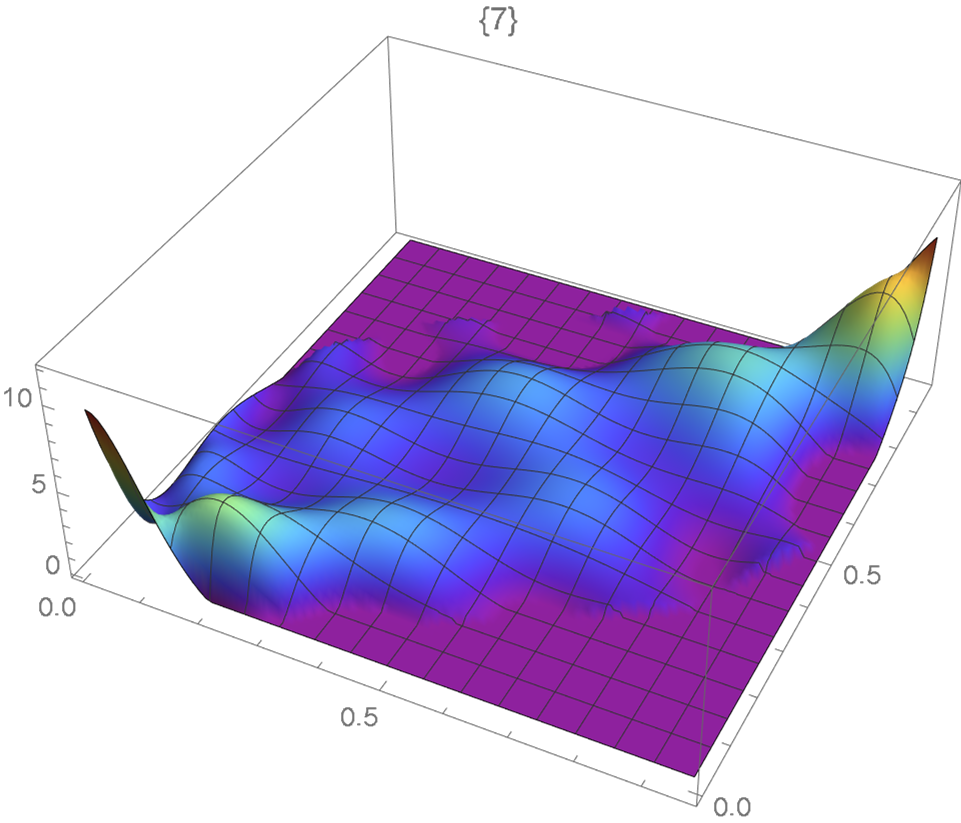
The resulting KDE based (Section 2) and moment-based (Section 3) copula densities plotted in Figures 6 and 7 are seen to be similar.
5.2. Determination of a Joint Density Estimate from the Marginal Density Estimates and the Copula Density
Using the two stocks’ standardized data, a Bernstein’s copula density of degree 250, which is shown in Figure 8, is utilized as an estimate of the underlying copula density. The density estimate (Figure 9) resulting from the application of the methodology described in Section 4 and a KDE (Figure 10) exhibit very similar features.
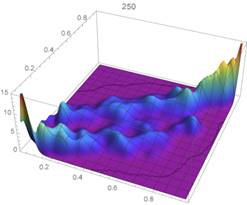

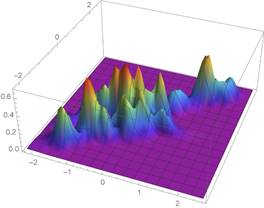
5.3. Copula Associated with a Brownian Motion Process and Its Running Maximum
The data consists of the daily closing prices of AC.TO (Air Canada) during 2019. To relate the data to a standard Wiener process, the first data point should be zero, the differences between successive observations should ideally often change signs and have a variance of one, and there should be one unit of time between successive observations. Hence the following transformation is used.
Let U1,U2,…,U_n denote the closing prices and V1,V2,…,Vn-1 be the differences between successive closing prices, that is, Vi=Ui+1-Ui; denoting by σD the standard deviation of the differences V1,V2,…,Vn-1, the following transformation is applied Wi=(Ui-U1)/σD and the resulting data is denoted by W1,W2,…,W_n.
Let Zi be the ith running maximum, that is, Zi="Max"\{W1,W2,…,Wi \}, i=1,2,…,n. Then, the resulting bivariate data, (Wi,Zi ), i=1,2,…,n, has the features of a Brownian motion process and its running maximum.
The Wi’s and Zi’s are plotted in Figures 11 and 12. The resulting copula density as obtained by applying the methodology described in Section 2, is shown in Figure 13.
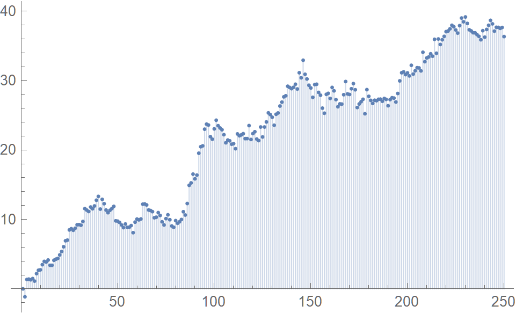
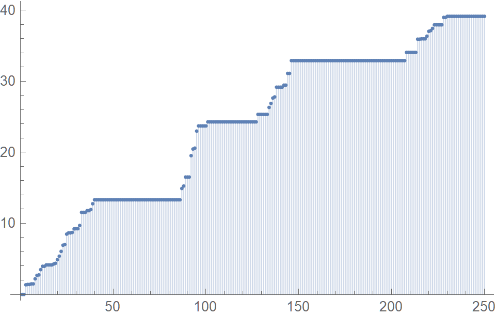
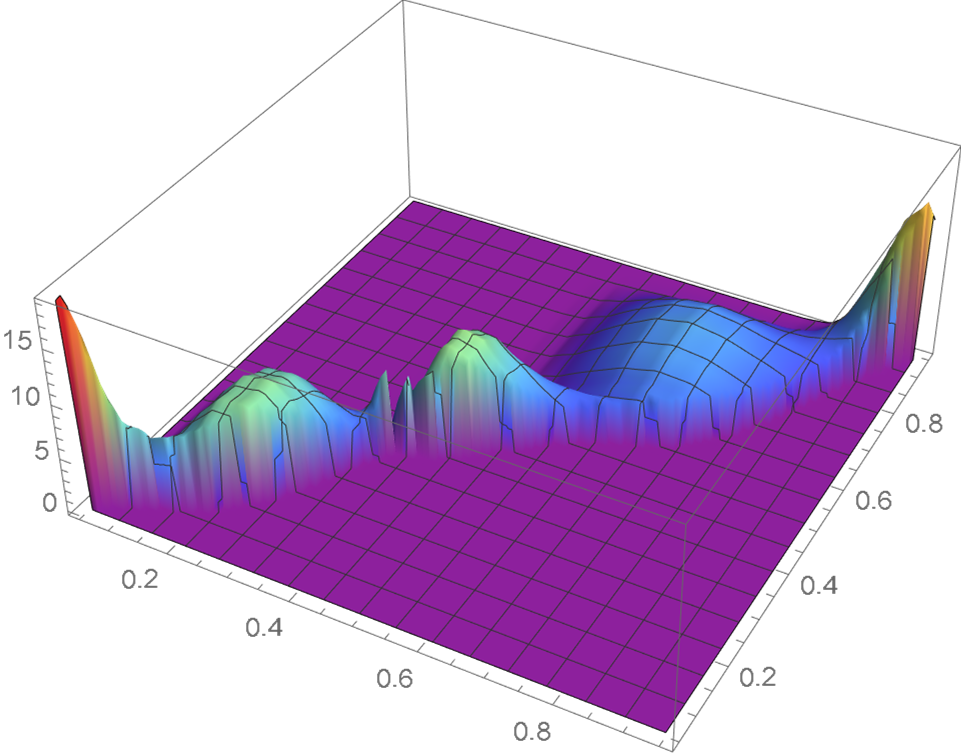
6. Conclusion
As copula density estimates are usually expressed in complicated forms, the bivariate polynomial approximation that is proposed in this paper ought to prove more suitable for reporting purposes. Approximations by means of Bernstein polynomials and the kernel density estimation approach are discussed as well. Additionally, a flexible technique for estimating joint density functions is introduced. The proposed methodologies were successfully applied to two stocks' closing prices as well as a set of observations and its running maximum.
References
[1] M. Chao, S. Z. Xin, and L. S. Min, "Neural network ensembles based on copula methods and distributed multiobjective central force optimization algorithm," Engineering Applications of Artificial Intelligence, vol. 32, pp. 203-212, 2014. View Article
[2] D. Carrera, R. Santana, and J. A. Lozano, "Vine copula classifiers for the mind reading problem," Progress in Artificial Intelligence, vol. 5(4), pp. 289-305, 2016. View Article
[3] T. Chen, S.-S. He, J.-Q. Wang, L. Li, and H. Luo, "Novel operations for linguistic neutrosophic sets on the basis of Archimedean copulas and co-copulas and their application in multi-criteria decision-making problems," Journal of Intelligent & Fuzzy Systems, vol. 37(2), pp. 2887-2912, 2019. View Article
[4] M. Sklar, "Fonctions de répartition à n dimensions et leurs marges," Publications de I'Institut de statistique de I'Université de Paris, vol. 8, pp. 229-231, 1959.
[5] A. Sancetta and S. Satchell, "The Bernstein copula and its applications to modelling and approximations of multivariate distributions," Econometric Theory, vol. 20, pp. 535-562, 2004. View Article